What is Linear Regression Analysis?
- Data Investigator Team
- May 22, 2023
- 3 min read
Updated: Jun 13, 2024
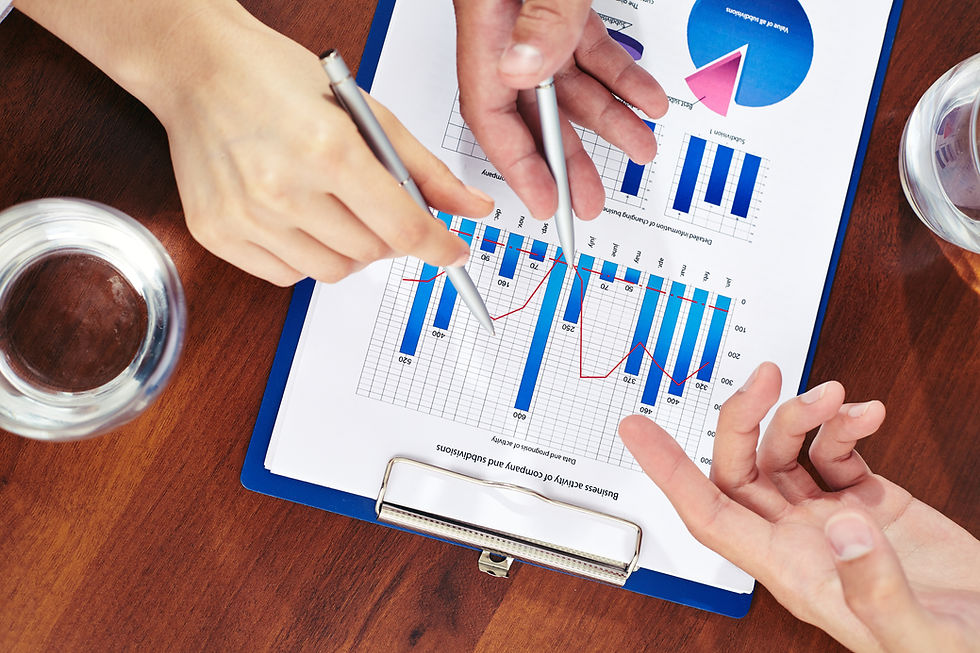
What is Linear Regression Analysis?
Linear regression is a statistical method used to analyze the relationship between one or more independent variables and a dependent variable. Simple linear regression involves a single independent variable, while multiple linear regression involves two or more independent variables. This article will explain what simple linear regression and multiple linear regression are and illustrate the examples of the uses and the limitations.
1. Simple Linear Regression
1.1 What is Simple Linear Regression:
Simple linear regression is used to model the linear relationship between a dependent variable (y) and a single independent variable (x). It assumes a linear relationship between the variables, meaning that the relationship can be represented by a straight line. The goal of simple linear regression is to find the equation of the line that best describes the relationship between the variables.
1.2 When to Use Simple Linear Regression:
Simple linear regression is used when there is reason to believe that a single independent variable is related to the dependent variable. It is often used in business and economics to analyze the relationship between two variables, such as the relationship between advertising expenditures and sales. It is also commonly used in scientific research to analyze the relationship between a single predictor variable and an outcome variable.
1.3 Examples of Hypotheses:
A simple linear regression model can be used to test various hypotheses. For example, in a business context, a hypothesis may be that an increase in advertising expenditures leads to an increase in brand awareness level. The simple linear regression model can be used to test this hypothesis by analyzing the relationship between years of experience (the independent variable) and the increase in salary (the dependent variable).
1.4 Limitations:
Simple linear regression has limitations that must be considered when interpreting the results. One limitation is that it assumes a linear relationship between the variables, meaning that the relationship can be represented by a straight line. If the relationship is nonlinear, simple linear regression may not be appropriate. Another limitation is that the model assumes that the error terms are normally distributed. If the error terms are not normally distributed, the results may be inaccurate.
2. Multiple Linear Regression
2.1 What is Multiple Linear Regression:
Multiple linear regression is used to model the linear relationship between a dependent variable (y) and two or more independent variables (x1, x2, x3, etc.). It assumes a linear relationship between the variables, meaning that the relationship can be represented by a straight line. The goal of multiple linear regression is to find the equation of the line that best describes the relationship between the variables.
2.2 When to Use Multiple Linear Regression:
Multiple linear regression is used when there is reason to believe that multiple independent variables are related to the dependent variable. It is often used in business and economics to analyze the relationship between multiple variables, such as the relationship between advertising expenditures, price, and sales. It is also commonly used in scientific research to analyze the relationship between multiple predictor variables and an outcome variable.
2.3 Examples of Hypotheses:
A multiple linear regression model can be used to test various hypotheses. For example, in a business context, a hypothesis may be that an increase in advertising expenditures and a decrease in price leads to an increase in sales. Another example can be that advertising expenditure, website traffic and social media engagement significantly influence the product sales.
2.4 Limitations:
Multiple linear regression also has limitations that must be considered when interpreting the results. One limitation is that it assumes a linear relationship between the variables, meaning that the relationship can be represented by a straight line. If the relationship is nonlinear, multiple linear regression may not be appropriate. Another limitation is that the model assumes that the error terms are normally distributed. If the error terms are not normally distributed, the results may be inaccurate. Additionally, multicollinearity can occur when the independent variables are highly correlated, which can lead to unstable estimates and inaccurate results.
For more information, please kindly contact:
Line Official Account: @datainvestigatorth
Tel: 063-969-7944
E-mail: info@datainvestigatorth.com
Comments