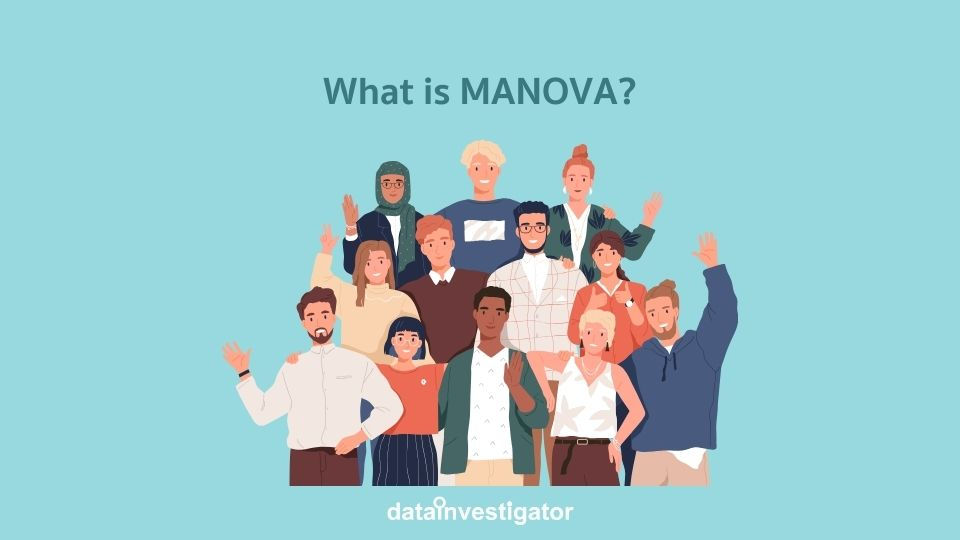
MANOVA, or Multivariate Analysis of Variance, is a statistical technique used to analyze data with multiple dependent variables simultaneously. It examines whether there are significant differences in the dependent variables among different groups defined by the independent variables.
When to Use MANOVA?
MANOVA is suitable for situations where:
Multiple Dependent Variables: When there is more than one dependent variable that may be related.
Independent Variables: When there is one or more independent variables that categorize the data.
Analyzing Overall Impact: When you want to analyze the impact of the independent variables on multiple dependent variables simultaneously.
Characteristics of Variables
Dependent Variables: Should be continuous and normally distributed.
Independent Variables: Should be categorical variables that divide the data into groups.
How MANOVA Differs from Multiple ANOVAs
Using MANOVA differs from using multiple ANOVAs in several ways:
Considering Relationships Among Dependent Variables: MANOVA considers the relationships among dependent variables, which multiple ANOVAs do not. Ignoring these relationships can lead to incorrect conclusions.
Testing Overall Effects: MANOVA tests the overall effects of the independent variables on the dependent variables simultaneously, whereas multiple ANOVAs test each dependent variable separately.
Reducing Type I Error: MANOVA reduces the chances of making a Type I Error (false positive) compared to running multiple ANOVAs.
What is a Type I Error?
A Type I Error occurs when the null hypothesis is incorrectly rejected, meaning a difference is concluded when there is none. For example, concluding that a treatment is effective when it actually is not.
Research Examples Using MANOVA
Example 1: Studying the effects of different training programs on employee performance indicators.
Independent Variable: Type of training (Training A, B, C)
Dependent Variables: Job performance, job satisfaction, stress level
Hypotheses:
H0: The type of training has no effect on job performance, job satisfaction, and stress level.
H1: The type of training affects job performance, job satisfaction, and stress level in at least one variable.
Example 2: Investigating the effects of different learning technologies on educational success indicators.
Independent Variable: Type of learning technology (Online learning, Application-based learning, Classroom learning)
Dependent Variables: Exam scores, content comprehension, learning satisfaction
Hypotheses:
H0: The type of learning technology has no effect on exam scores, content comprehension, and learning satisfaction.
H1: The type of learning technology affects exam scores, content comprehension, and learning satisfaction in at least one variable.
Interpreting MANOVA Results
After conducting MANOVA, key results to consider include:
Pillai’s Trace, Wilks’ Lambda, Hotelling’s Trace, Roy’s Largest Root: These statistics test for differences between groups. If the p-value is less than 0.05, it indicates significant differences.
Post Hoc Tests: If MANOVA shows significant differences, follow-up analyses such as ANOVA for each dependent variable or pairwise comparisons should be performed.
Effect Sizes: Use measures like Partial Eta Squared to assess the magnitude of the differences.
Example Interpretation
If the MANOVA results show a significant Wilks’ Lambda (p < 0.05), it indicates that the type of exercise has a collective impact on weight, blood pressure, and blood sugar levels. Further analysis may reveal:
Weight: Significant difference between Exercise A and B.
Blood Pressure: No significant difference.
Blood Sugar Levels: Significant difference between Exercise A and C.
MANOVA is a powerful statistical tool for examining the impact of independent variables on multiple dependent variables simultaneously. It provides a more comprehensive analysis and reduces the chances of Type I Errors compared to multiple ANOVAs. For more detailed inquiries or assistance with MANOVA, please kindly contact our experts.
For more information, please kindly contact:
Email: info@datainvestigatorth.com
Line: @datainvestigator
Comments